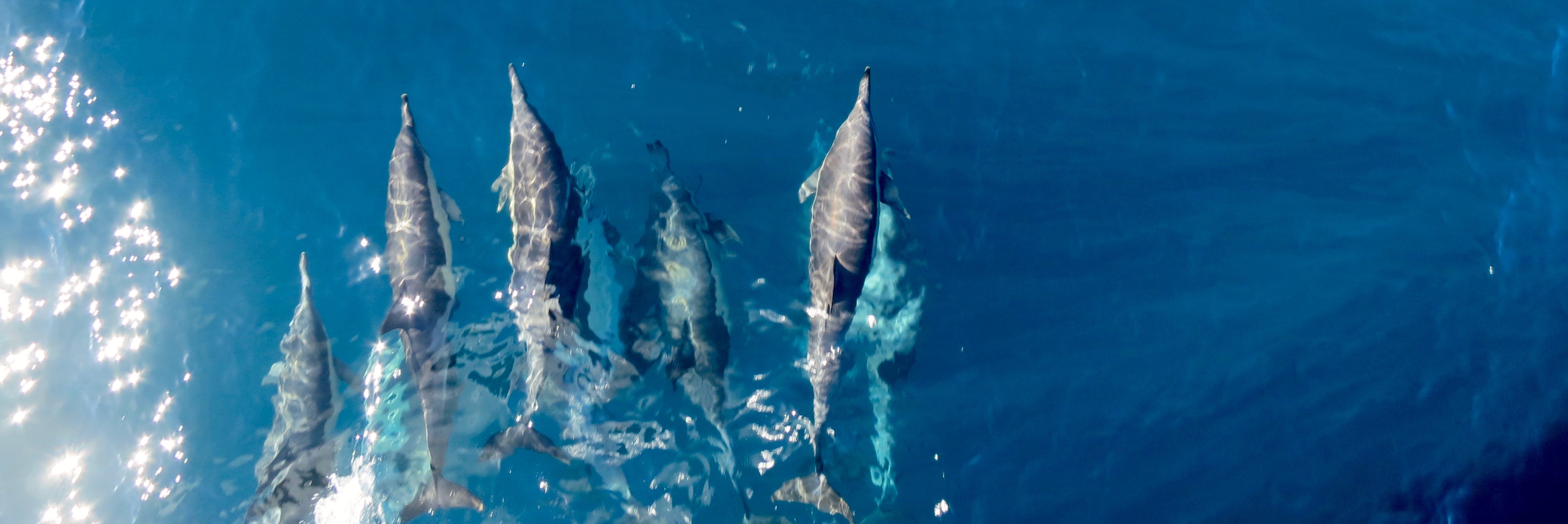
Photo : The bow of the RS Algoa in the Agulhas Current, Indian Ocean, April 2016
Projects
Towards two decades of ocean mass and heat transports at 26.5°N: Extension of the RAPID-MOCHA Array
I am the co-PI of this project led by PI Bill Johns from the Rosenstiel School of Marine and Atmospheric Science at the University of Miami. Climate models suggest that variability of the Atlantic meridional overturning circulation (AMOC) is linked to sea surface temperature changes on interannual to multi-decadal time scales that can have far reaching societal impacts. Additionally, climate models continue to project a substantial weakening of the AMOC over the next century that could feed back on the climate system through reduced northward heat transport as well as reduced CO2 uptake in the North Atlantic. The specific role of the AMOC in the climate system has been difficult to determine due to a lack of long term measurements of its variability. At the end of its current phase, the RAPID-MOCHA observing system along 26.5°N will have provided continuous estimates of the AMOC for just under 19 years, the longest full-depth, basinwide measurements of oceanic transport currently available. These observations have provided a critical benchmark for the evaluation of forced, data-assimilative, and coupled climate models, and have formed a fundamental building block around which international programs are being developed to monitor the AMOC throughout the Atlantic basin.
This project continues the U. S. contribution to RAPID-MOCHA from January 2023 to June 2026, extending the time series of oceanic mass, heat, and freshwater transports to 22.3 years. The U.S. contribution to the in-situ observation system includes: (1) Florida Current monitoring using a submarine cable, calibrated by regular cruises with hydrographic and velocity measurements, and (2) continuous moored time series measurements within the Deep Western Boundary Current and Antilles Current region east of the Bahamas, including hydrographic and velocity sections at approximately 11-month intervals. The maintenance of the repeat hydrographic sections is conducted in collaboration with NOAA which also supports independently the Florida Current monitoring. The U.K. National Oceanography Centre provide support for western and eastern basin moorings that complete the 26.5°N trans-basin time series observing system. As part of this new iteration of RAPID-MOCHA, limited new instrumentations on moorings will also be included to derive ocean bottom pressure gradient on the boundary east of the Bahamas. Overall, the RAPID-MOCHA observations will allow us to document and understand the causes and impacts of the AMOC interannual to decadal variability, to investigate the control of western boundary pressure on transports, and to link changes observed at 26.5°N to other AMOC indices and results from on-going AMOC measurement programs around the Atlantic basin.
EarthCube Capabilities: CloudDrift: A platform for accelerating research with Lagrangian climate data
I am the lead PI of the CloudDrift project which aims at easing and accelerating the use of Lagrangian data for atmospheric, oceanic, and climate sciences. Utilizing the data from the NOAA Global Drifter Program as a working framework, we will design a set of open source software tools for processing, formatting, and analyzing Lagrangian data within cloud storage and cloud computing environments (that is, making these dataset analysis-ready and cloud-optimized). Targeting users ranging from high school students to climate and statistical experts, we will build these tools in modular ways so that they can be portable and adaptable to other datasets derived from observations and numerical models. The geosciences have entered a new phase of open science for which advances in data format and availability, software modularity and portability, and on-demand distributed cloud computing are not only accelerating research workflows but also triggering new data-oriented and curiosity-driven investigations. By creating new analysis-ready and cloud- optimized datasets of essential climate variables gathered by drifters at the air-sea interface, the CloudDrift project will not only add scientific value for climate research to the NOAA GDP, but also increase the discoverability of its datasets. By bringing these data and their derived and portable analysis tools to cloud computing environments within proximity of other major climate datasets, we envisage that this will allow the newly cognizant communities to systematically interrogate with some of the latest machine learning and data mining tools relationship patterns between climate variables in order to trigger data-led discoveries. The Pangeo community platform is a partner for this project. The funding for this project is provided by EarthCube, a partnership between the NSF Directorate for Geosciences (GEO) and the NSF Office of Advanced Cyberinfrastructure (OAC) in the Directorate for Computer & Information Science & Engineering (CISE).
Applying machine learning tools to investigate oceanic heat transport and ocean currents and temperatures predictions
The climate of the Earth is characterized by a marked difference of surface temperature between the Equatorial region and the polar regions. This temperature difference would only be greater if it were not tampered by the continual redistribution of thermal energy, or heat, orchestrated by both the atmosphere and the ocean. Despite the oceanic heat transport being so important, the exact mechanisms by which this one is conducted are not completely understood. Furthermore, how the ocean heat transport plays along with its atmospheric counterpart adds complexity. One difficulty is the turbulent nature of ocean currents, which at times can obfuscate the simplified picture of heat transport just described. Practically, heat transport at the ocean surface manifests itself as the covariance between the sea surface current (SSC) and the sea surface temperature (SST): a northward, positive SSC anomaly associated on average with a warm, positive anomaly of SST corresponds to a positive covariance—or positive correlation—and therefore a northward oceanic heat transport. And vice versa: a negative correlation corresponds to a southward transport. As such, determining in time and space the correlation patterns between SSC and SST could provide a mapping of oceanic heat transport, and inform us on one of the most primordial mechanisms of our climate. At any rate, such processes can be studied with ocean models, but the latest and state-of-the-art models are dramatically in need of being benchmarked by observations. Using a unique in situ dataset of SSC and SST, we will systematically interrogate with machine learning tools the patterns of correlations between these two variables in order to ultimately unravel the mechanisms of oceanic heat transport. I am leading this project with Melvin Ayala (University of Miami). The funding is provided by the University of Miami Institute for Data Science and Computing (IDSC) through the IDSC grant Expanding the Use of Collaborative Data Science.
A Pilot Project: Measuring mean sea level with surface drifting buoys
The overarching goal of this pilot project is to investigate the capability of the drifting buoys (hereafter drifters) of the Global Drifter Program (GDP) to measure mean sea level (MSL) and eventually global MSL (GMSL) and its changes. The GDP has now almost entirely transitioned to equipping its drifters with GPS receivers for positioning, from previously using the Argos system. This opens the possibility of not only acquiring highly accurate latitude and longitude of drifters at sea but also their altitude or geocentric height above the center of the Earth, at hourly, sometimes more frequent, time steps. Once a reference mean sea surface is subtracted from GPS height, a measure of sea level should be obtainable by a drifter. In an proof-of-concept (Elipot 2020), a numerical simulation shows that the sampling pattern of the drifter array is adequate to measure with sufficient accuracy the GMSL and its changes. This proposed enhancement of the existing observing system could augment the on-going satellite and tide gauge observing systems for GMSL monitoring. In addition, in case of interruption of the satellite measurements, this new system could prevent gaps in the historical records. This limited pilot project aims at determining the necessary modifications of a drifter’s hardware to meet the required GPS height accuracy for MSL measurements. This is a pre-requisite for proposing a global implementation of this new ocean observing system for global mean sea level, a concept selected as an “Ocean-Shot” concept by the U.S. National Committee for the United Nations Decade of Ocean Science for Sustainable Development Elipot et al. 2021. The funding for this project is provided by NASA JPL.
Mapping the kinematics and dynamics of tidal ocean currents with surface drifters
I am the lead-PI of this NSF-funded project, in collaboration with Ed Zaron from Oregon State University, and Rick Lumpkin from the NOAA Atlantic Oceanographic Meteorological Laboratory. This project will generate new knowledge concerning tidal currents in order to better understand tidal dynamics and their role in the maintenance of the global thermohaline ocean circulation. The drifter dataset that will be utilized to investigate baroclinic tidal phenomena is both large enough and unique (in terms of time span, geographic coverage, and accuracy) to provide a quantitative description of tidal properties. In addition, qualitative and potentially new assessments of tidal/non-tidal interactions will be obtained, including their seasonal and longer temporal variability. A novel decomposition of the tidal energy budget, which separates processes primarily affecting phase-locked and non-phase-locked tidal signals, is proposed to guide the analysis and interpretation of not-only the drifter observations, but also observations from altimetry and numerical simulations which will be used alongside the drifter data.